Feature: AI, the new research partner
Algorithms can fix it
It’s a simple fact that AI is being used increasingly in research. We look at six current projects using AI in the hope it can produce special insights.
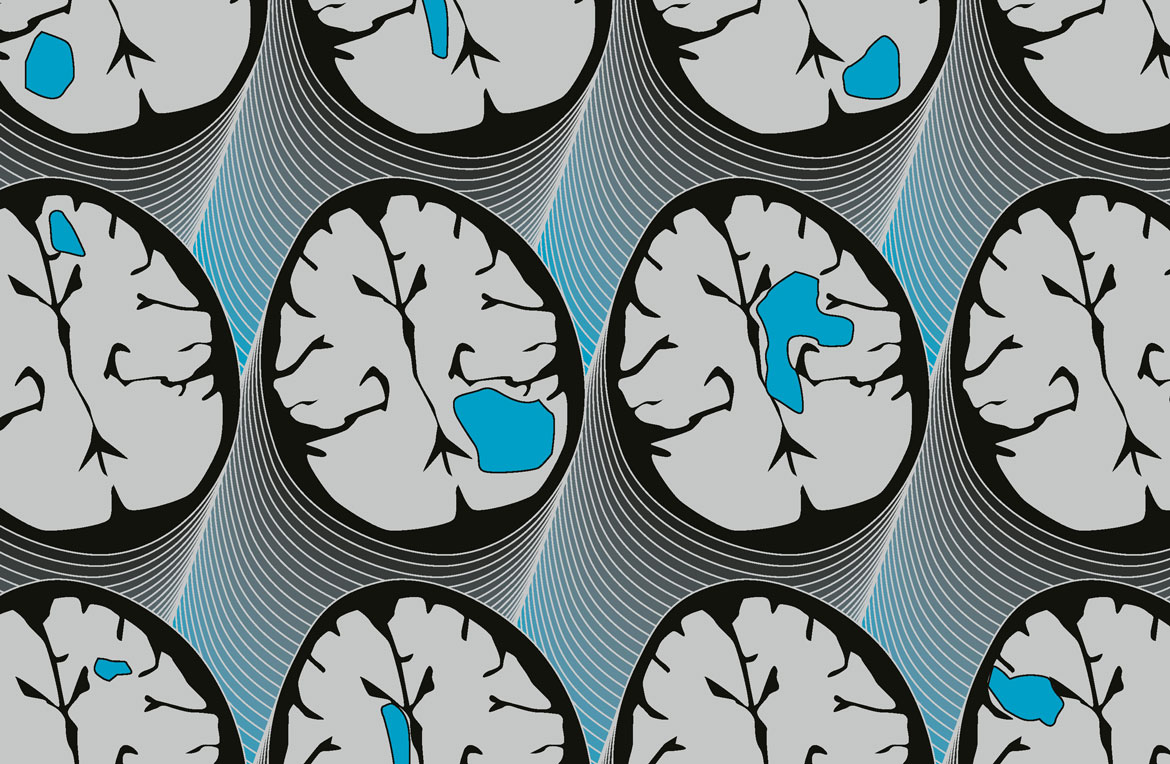
Getting rid of a malignant tumour
If you’re going to treat fast-growing brain tumours successfully, you need to make decisions swiftly. To this end, doctors rely on MRI images. For ten years now, Mauricio Reyes at the ARTORG Center of the University of Bern has been researching into an especially aggressive type of tumour called glioblastoma. Since last year, he has been using an AI system to predict the growth of these tumours. It is able to recognise different components of the tumour, and it’s hoped that it will be able to use image analysis to distinguish between tumours that are aggressive and those that are less active. The average prognosis for survival in the first instance is 16 months, but several years in the second. Being able to differentiate between them is therefore crucial when doctors are deciding what therapies should be used. “AI systems can primarily help us to win time”, says Reyes.
The first step he took when training his AI was to get the system to learn how to divide up the scan of the tumour into different areas: there are dead regions, active zones where the cancer cells are multiplying rapidly, and marginal regions where inflammatory processes are dominant. He hoped that the AI would use the development of these zones to learn how to distinguish variants with different rates of aggression. And it was successful: the AI was able to predict different variants of the tumour to an accuracy of 88 percent. “The problem was that we didn’t know exactly how the AI made its decisions”, says Reyes. “We are often dealing with ‘black-box’ systems”.
Reyes wanted to understand what pixels the AI was using for its decision-making. “We found that it was looking mainly at the peripheral regions of the tumour, not at the active zones. That was wrong”. Researchers call this phenomenon ‘shortcut learning’. This is where AI systems make statements that are more or less correct, but for the wrong reasons. If you rely blindly on black-box systems, especially in the medical field, the consequences can be fatal. Reyes has now excluded the peripheral regions of the tumour when getting the AI to make its evaluations, because, just like the dead regions, the periphery of the tumour can provide almost no information at all. Once Reyes had done this, the accuracy of his AI’s predictions rose to 98 percent. He is convinced that there is an urgent need to develop an AI for the future that can control the quality of other AIs. As a result, his next project is called: ‘AI for AI’. Up to now, no such thing exists.
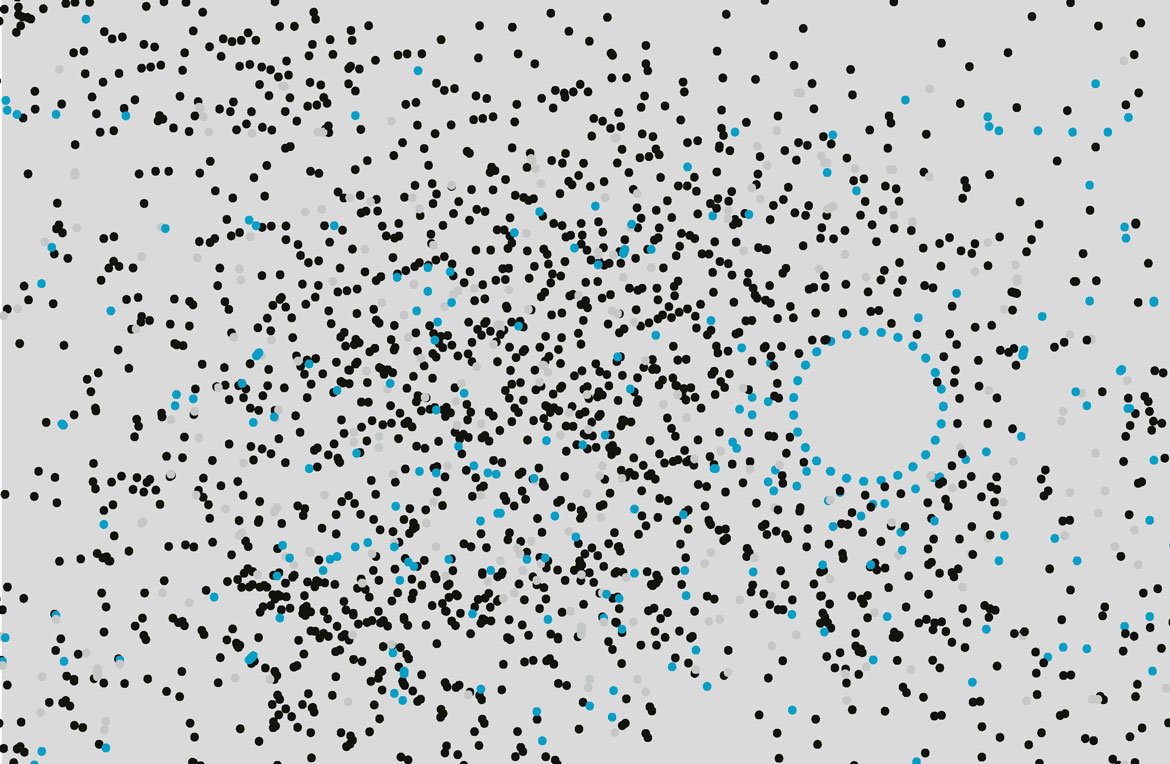
The giddy noise of a potential new physics
When the Higgs boson was discovered at the Large Hadron Collider (LHC) at CERN in Geneva, it provided complete proof for the Standard Model of physics. Now physicists understand the electromagnetic force, the strong force and the weak force. However, our description of the Universe remains incomplete, because the Standard Model can’t explain phenomena such as dark energy and dark matter. It is therefore highly likely that other particles exist. A research team led by Steven Schramm at the University of Geneva believes that they can find clues to undiscovered particles in the abundant low-energy collisions produced at the LHC. They now want to use AI to help them take so-called data noise – which is caused by overlapping signals, inaccurate measurements and equipment errors – and transform it into actual data so that patterns might be recognised in it. Such a pattern could point to a particle that would explain the origin of dark matter. “We hope to discover new physics”, says Schramm.
It’s only with the help of AI that Schramm and his team will be able to process the huge amounts of data being gathered from the LHC detectors, he says. Overall, their task is challenging because it involves trying to understand a 3D image of the electrical signals generated by a particle collision. They are using so-called graph neural networks (GNNs) for this. They train their system with simulations from the Standard Model of particle physics, which teach it to recognise normal events. Once they’ve done this, they hope that their AI will be able to find deviations in the data noise of real collisions, i.e., indications of a possible new physics. At the same time, the AI has to be able to rule out expected anomalies – errors made by one of the LHC detectors.
“Using AI to help in our search for anomalies is a completely new approach”, says Schramm. “It means we can undertake an efficient hunt for phenomena that have not been predicted by the theory”. And what if their search fails? Then at least Schramm & Co. will have found swifter proof that there is no new physics hidden away in the energy ranges they are investigating.
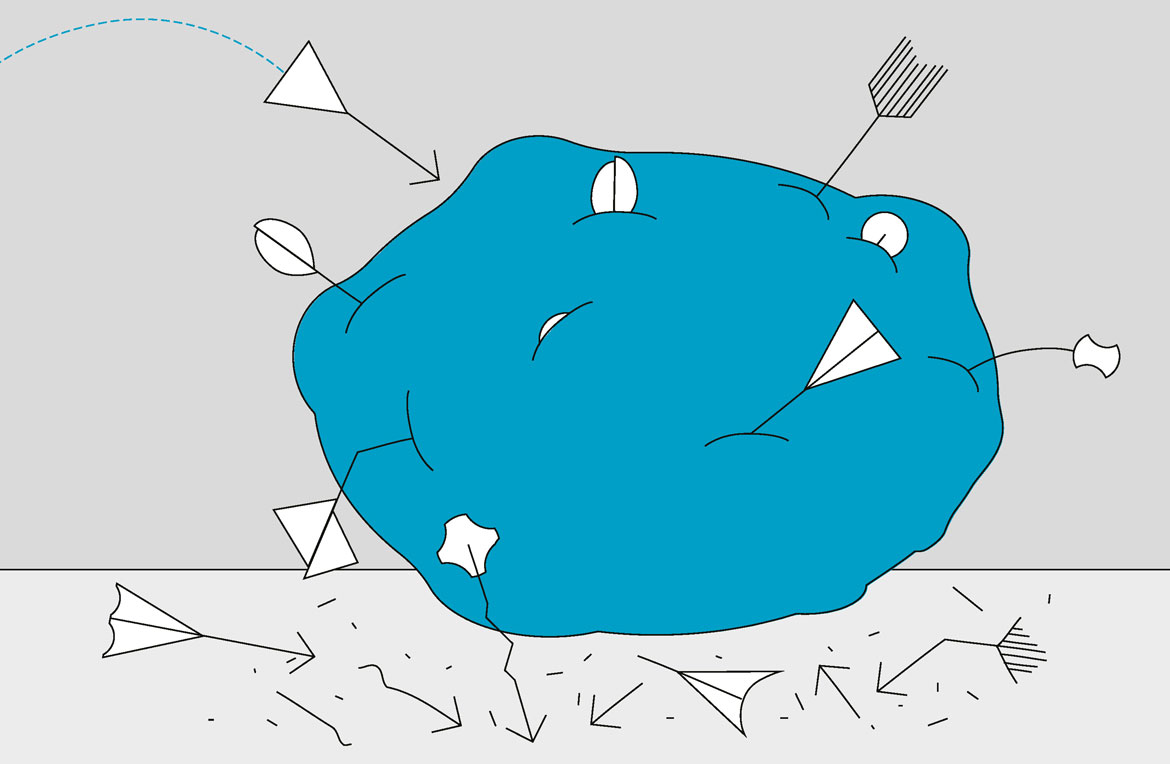
Helping a drug to penetrate a cell
One of the biggest challenges when developing novel drugs is how to ensure that the therapeutically active molecules reach their destination, especially when the target structures are located inside cells. Every cell is surrounded by a boundary layer: a protective biomembrane that also mediates its contact with the outside world. So it is incredibly important for us to understand the behaviour of so-called cell-penetrating peptides (CPPs). These short amino acid sequences can penetrate a cell membrane and channel molecules into cells. “They offer us great potential for targeted drug delivery in future”, says Gianvito Grasso of IDSIA.
Since conventional methods for predicting the behaviour of these peptides are either experimentally challenging or demand high-intensity computing to create the necessary models, the IDSIA team is relying instead on artificial intelligence. Algorithms are now learning to analyse existing, large-scale databases of known cell-penetrating peptides, and are identifying new peptides that possess similar properties.
They trained their system using data from the scientific literature on CPPs and other peptides. This literature contains information such as the peptide sequence, their biophysical properties, and details on their ability to penetrate cell membranes. IDSIA’s machine-learning system then used this dataset to analyse the amino acid sequences of known peptides of both types (those that can penetrate cells, and those that can’t) in order to identify structures associated with peptides’ ability to penetrate cells.
Their goal was to make accurate predictions about the ability of different peptides to penetrate cell membranes. According to Grasso, “The algorithm is able to do more than just predict the behaviour of peptides. It can also suggest modifications to peptide sequences so as to help them to penetrate a cell more easily”. This tool will help them to assess peptides better before synthesising them.
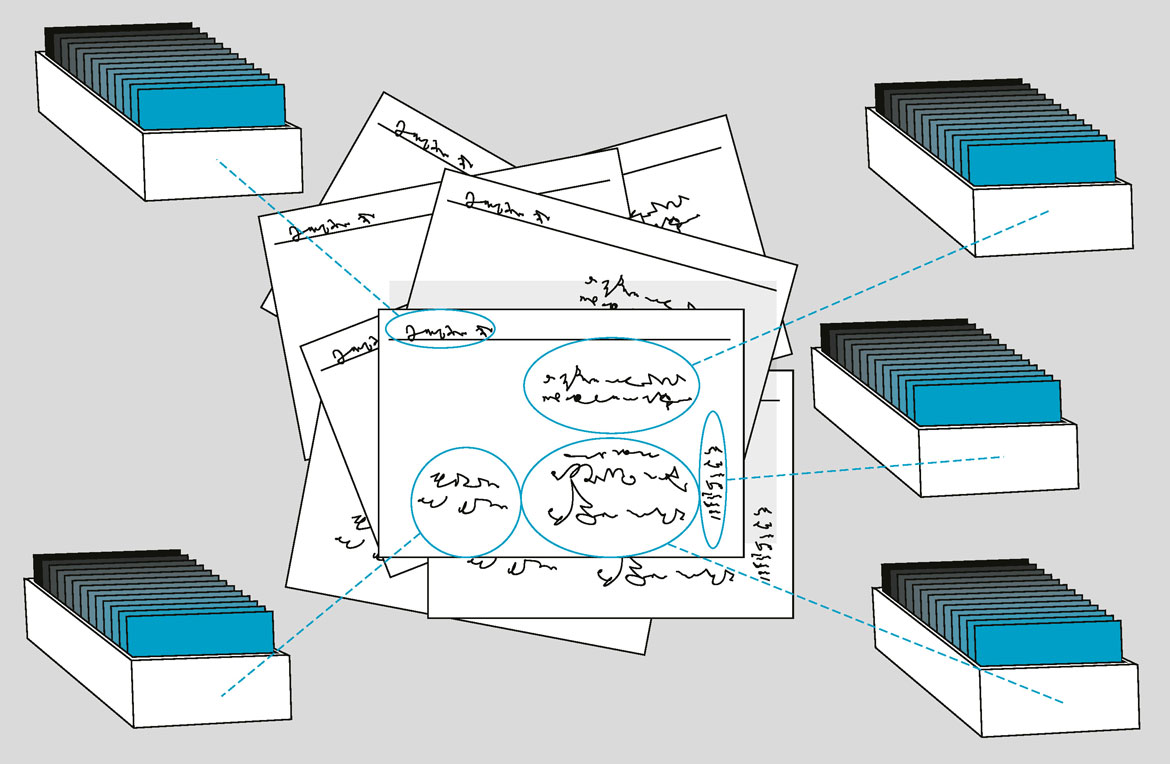
Ancient manuscripts under a digital magnifying glass
The historical land register of the city of Basel comprises more than 220,000 index cards holding information on the city’s buildings, all the way back to the 12th century. “Such a register is of immense value to historians”, says Lucas Burkart of the University of Basel. The problem is that its entries were written by hand. In order to make these index cards more easily accessible for people researching into the history of the city, Burkart and his colleague Tobias Hodel from the University of Bern have begun using AI-based indexing methods.
Their aim is to capture the information on these index cards and to evaluate it for a period of 300 years, from 1400 to 1700. Burkart and Hodel want to understand all the terms, specifications and customary procedures that applied to urban property in Basel, and to use this information to analyse shifts that occurred in the real estate trade, both in individual cases – e.g., the Klingental Convent – and for the entire city area.
Burkart and Hodel are using different machine-learning methods for this task, e.g., natural language processing (NLP). First, the system locates different text regions on the index card in spatial terms. Then it has to read the handwriting itself – recording the words in their respective language and handwriting type (including Latin script or an old German script). The third step is the most complex: “Here we break down each index card into so-called events”, says Hodel. By ‘event’ he means an action such as a purchase, a sale or a confiscation involving specific people or institutions, such as a monastery or convent. “These events are not always unambiguous and sometimes correspond to a peculiar historical logic that is not immediately comprehensible to us today”, says Burkart. “Different forms of economic transactions can be lurking behind a concept such as ‘interest’, for example”.
Their project also has an overarching goal. “We are trying to measure the error rate for each individual step of the AI’s evaluation process, such as how it records the different handwriting types”, says Hodel. Their prime concern is to determine the resilience of the results they are obtaining with their AI methods. Such analyses of the system, he says, “have to become part of the methodological development of the historical sciences”.
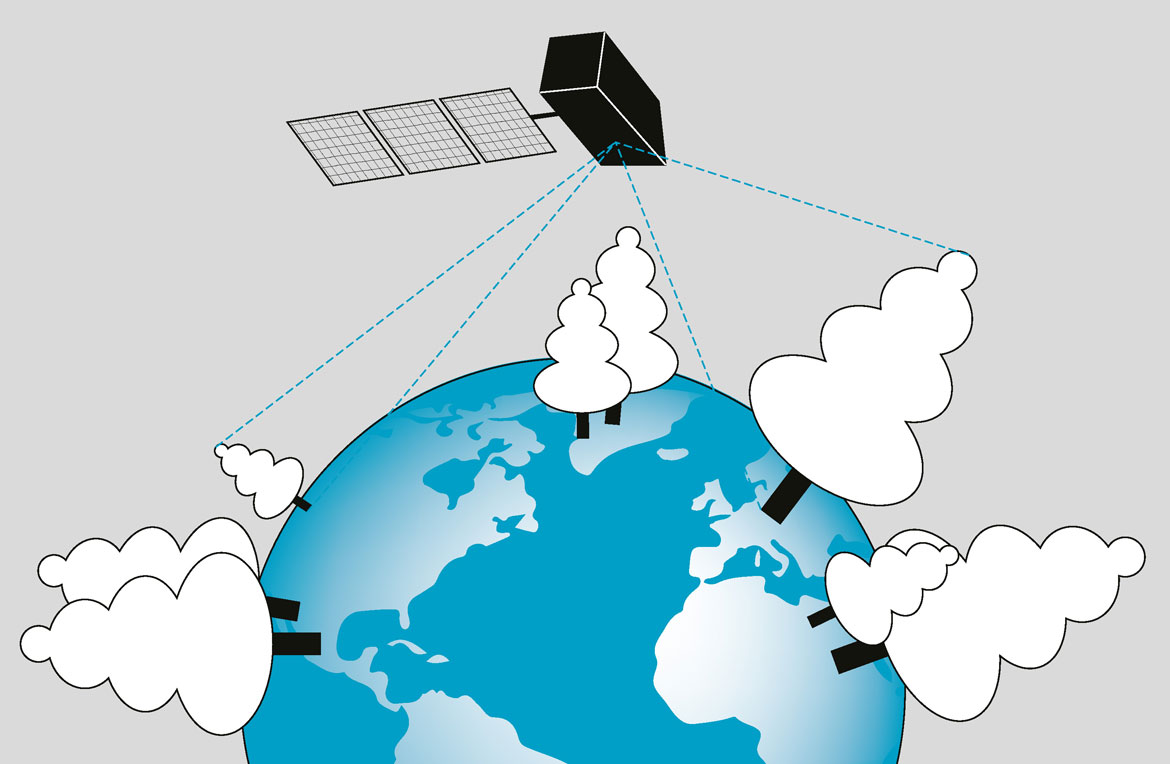
Viewing climate change from the treetops
At first glance, measuring the height of a tree doesn’t seem the kind of task that might require the assistance of artificial intelligence. But Konrad Schindler, a geodesist and computer scientist from ETH Zurich, doesn’t just want to measure a single tree. He wants to measure all the trees on Earth. Such data can be extremely significant because they can help him to create models for determining the distribution of biomass across the Earth – and thereby also assess the amount of carbon stored in its vegetation.
“We are the mapmakers for high-lying vegetation”, says Schindler. He is interested in whether AI methods can be used to help automate the mapping of environmentally relevant variables and correlations, e.g., vegetation density. This has until now been very difficult. “Our technology allows us to measure correctly even tall trees, which in terms of biodiversity and biomass are especially important”, he says.
This method is complicated, because the training data Schindler is using for his system are pixel images from cameras on the two ESA satellites Sentinel 2A and 2B. The reference data for training his system are provided by measurements of tree heights made by the GEDI laser scanner that NASA has mounted on the International Space Station. GEDI has made hundreds of millions of individual measurements up to now.
A physical model cannot be derived from the information in the pixels and the tree heights, as the connection between them is too complex, which is why Schindler is relying on machine learning. His system is based on a so-called deep neural network that is also able to indicate the statistical uncertainty of the results generated – essentially their degree of imprecision. Researchers call this ‘probabilistic deep learning’.
In the future, Schindler’s map could offer important guidelines for undertaking appropriate measures in the fight against climate change and against species extinction. For example, it could be used to monitor specific forest regions or protected areas. Here in Switzerland, conversations are already underway with the Federal Institute for Forest, Snow and Landscape Research (WSL).
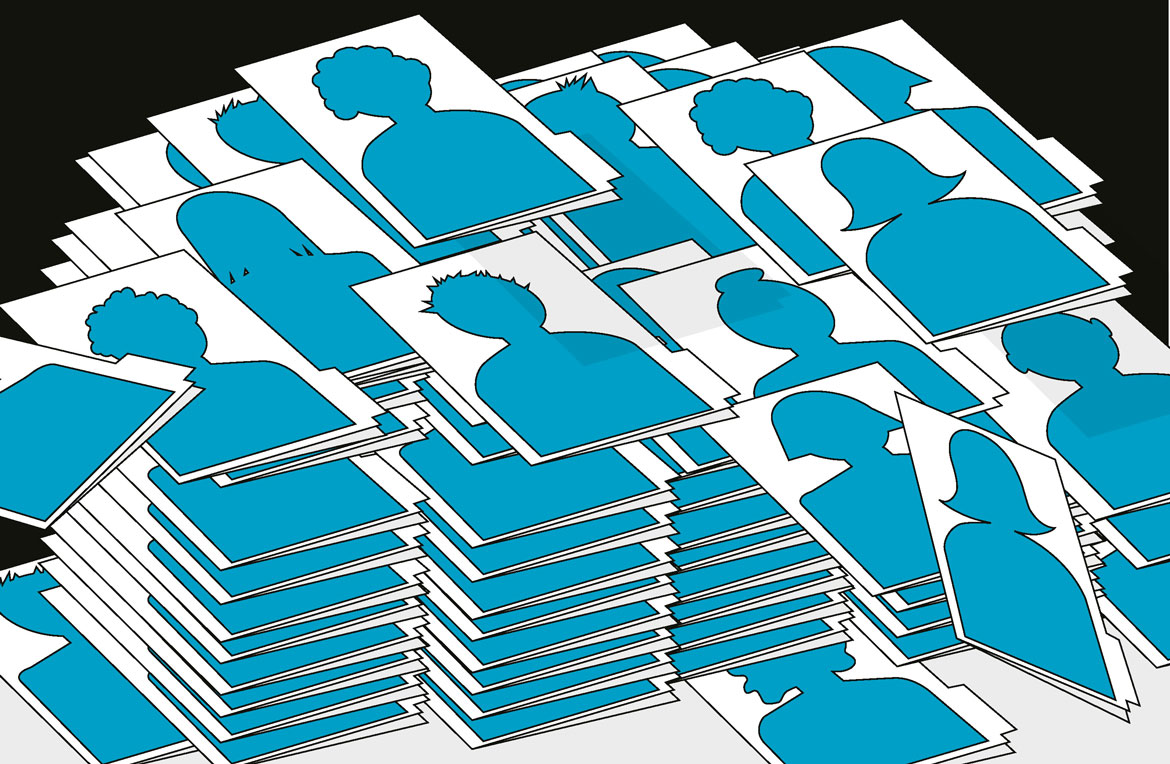
Progress with the anonymisation of health data
Elderly people are more likely to suffer from the side effects of medication, and are more susceptible to the unwanted interactions of different active agents. This is why a team at IDSIA in Lugano has been developing an AI that can detect possible complications at an early stage by automatically analysing the electronic medical records of elderly people in hospitals.
The data used for this are complex even for AI. Medical records often contain large chunks of text, and doctors in Switzerland also write their observations and diagnoses in the different national languages. What’s more, the data in these files are very unstructured. In order to be able to extract relevant information from them, the IDSIA team, led by Fabio Rinaldi, has been relying both on the technique of natural language processing (NLP), which is also used in systems such as ChatGPT that are a hot topic of debate right now, and on so-called text mining. Their aim was for their AI to recognise unwanted events and what triggers them.
In concrete terms, their project has been dealing with the side effects of anti-thrombosis drugs. For purposes of quality control, the AI used findings on interactions between different drugs from four Swiss hospitals that are involved in the study.
When using patient data for training purposes, anonymity is an important topic. The IDSIA team first had to apply appropriate NLP tools to anonymise all the medical records they wanted to use. Rinaldi believes that this anonymisation process for clinical reports has great potential, also above and beyond the scope of his own project. “We wanted to make valuable medical information available for scientific purposes”, he says. In general, he believes that when health-related data are brought together on a large scale, they can reveal medical information that is not visible when you consider only a few cases in isolation.
Illustrations: Anna Haas